Metacognition is all you need?
Using Introspection in Generative Agents to Improve Goal-directed Behavior
Jason Toy,
Josh MacAdam,
Phil Tabor
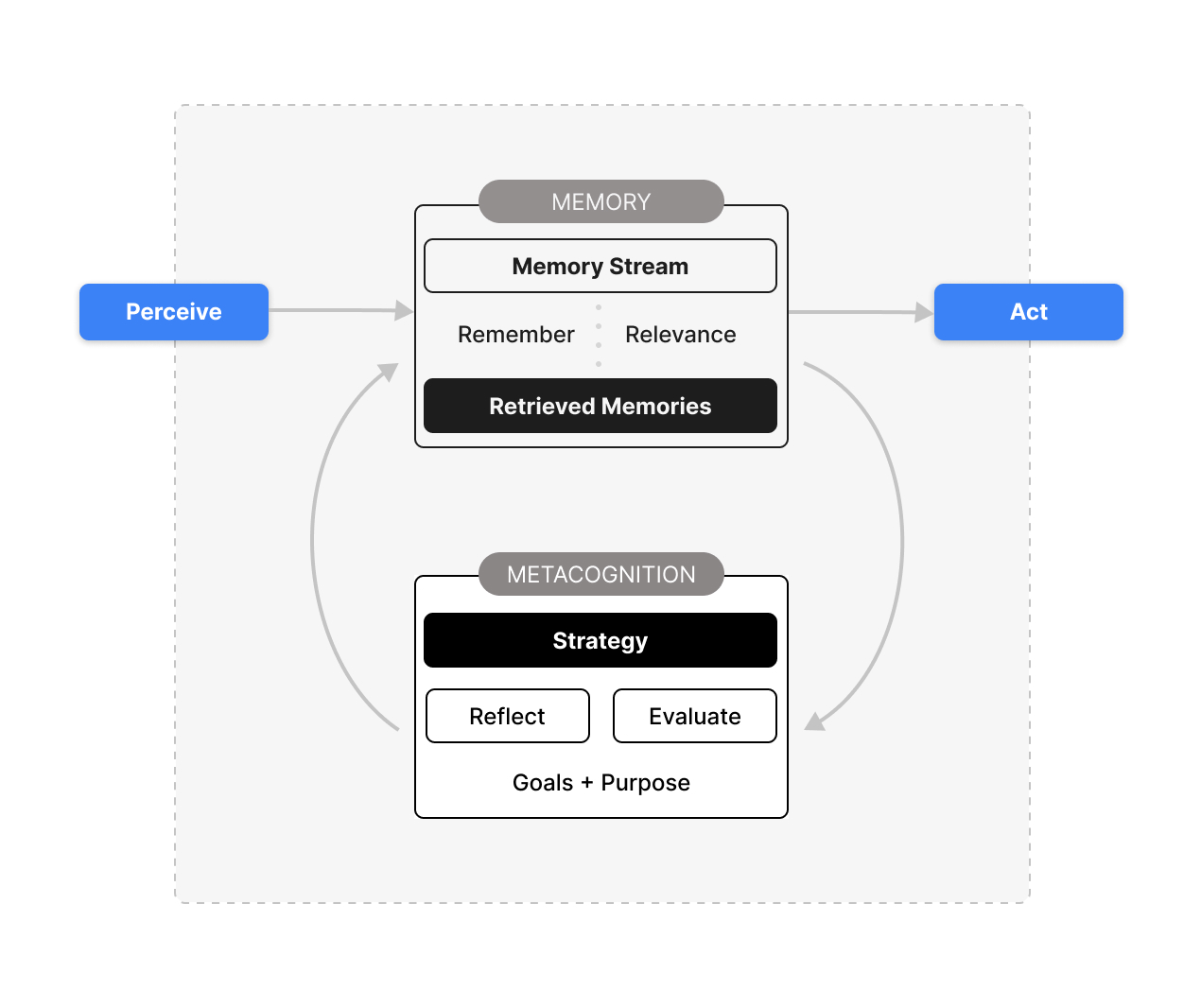
Overview
- LLMs are increasingly being used for agents with perpetual memories and chats
- Limited context lengths make LLM systems challenging to build
- An agent with a goal can easily get stuck on onforseen and unprogrammed situations
- An agent's strategy to achieve a goal must adapt to the situation over time, our framework allows for that
- A Metacognition module on top of an LLM allows goal oriented system to improve performance and adapt dynamically over time.
- Agents have a virtual memory external to an LLM that can grow beyond typical context lengths
- LLMs can be used to create dynamic interactive simulations with human-like belieavable agents
See Simulations
Citation
@article{toy2024metacognition,
title={Metacognition is all you need? Using Introspection in Generative Agents to Improve Goal-directed Behavior},
author={Jason Toy and Josh MacAdam and Phil Tabor},
year={2024},
journal={arXiv preprint arXiv:2401.10910}
}
Learn more about us